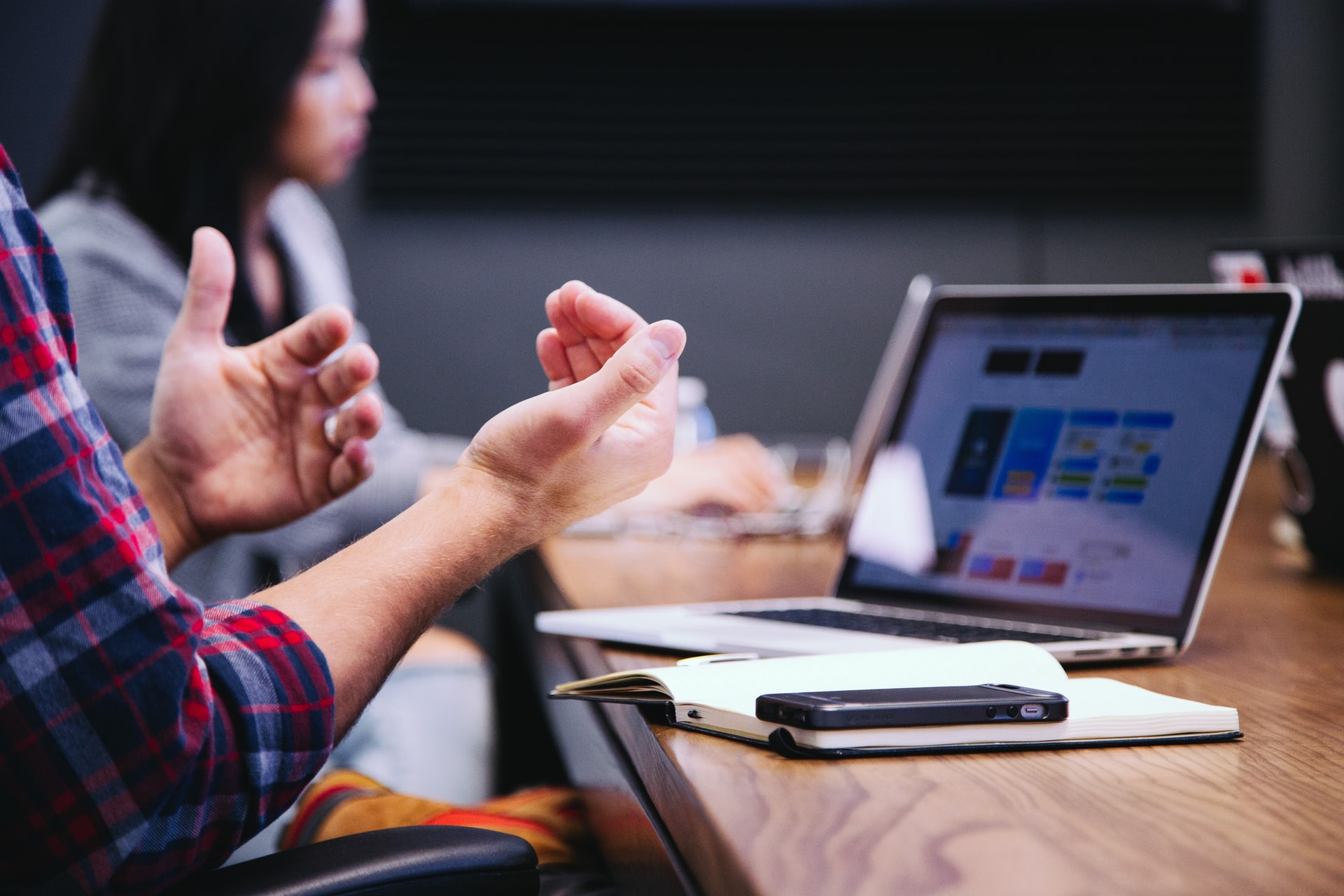
About
Speech, Audio and Language Processing are largely benefiting from deep learning architectures to achieve high levels of performance. Deep learning are the set of algorithms that allow to learn different levels of abstraction from given data. These algorithms have achieved great success in supervised environments. Supervised means that we have labelled data for training purposes. Deep learning algorithms typically need large quantities of labelled data to perform a task. Different architectures of these algorithms are combined and concatenated depending on the goal of the task. These can include recurrent neural networks that excel at modeling variable-length sequences, and convolutional neural networks that have typically been used to extract patterns from images. However, much more complex architectures such as the Transformer, which combine attention mechanisms and feed-forward networks, are so versatile that are able to succeed in multiple tasks. The purpose of this project is to focus on remaining challenges of advanced deep learning architectures in the context of speech, audio and language processing by continuing the intense research of our group. The project proposes to tackle big challenges in multilingual and multimodal machine translation, speaker recognition, natural language processing and speech regeneration.
Funded by the Spanish Ministerio de Ciencia e Innovación, the Agencia Estatal de Investigación through the project PID2019-107579RB-I00 (agreement AEI/10.13039/501100011033)
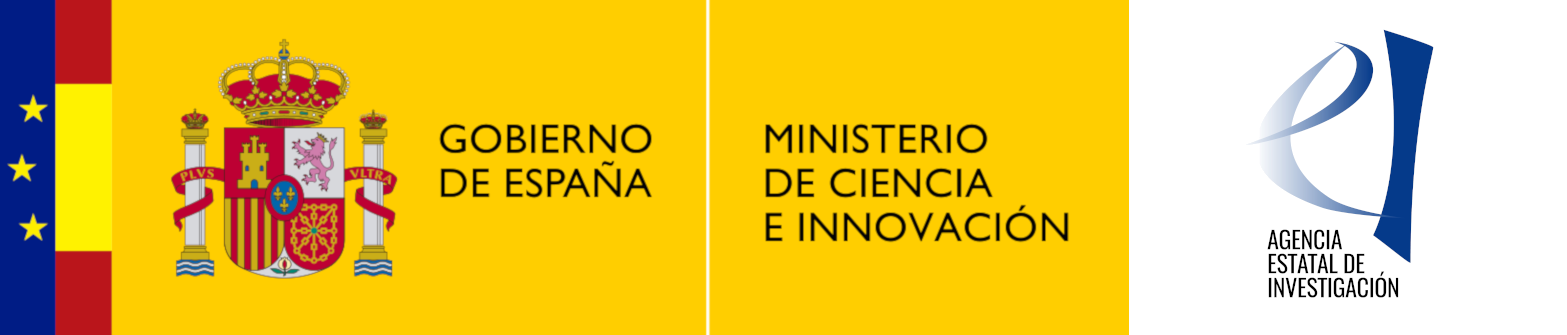
Principal Investigators: José A. R. Fonollosa & Marta R. Costa-jussà